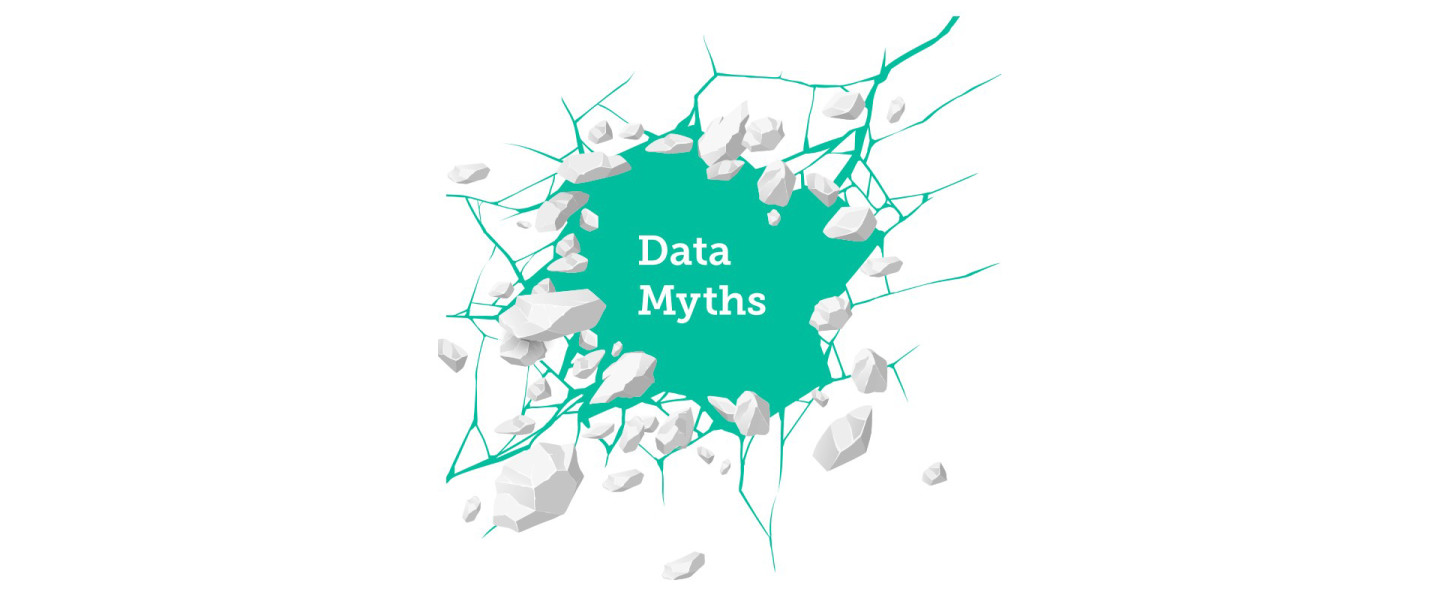
4 Data Myths Holding Back
Data Myths
Data is exploding. The volume of data generated, consumed, copied, and stored is projected to reach more than 180 zettabytes by 2025. In 2020, the total amount of data generated and consumed was 64.2 zettabytes. (Source: Statista)
The tremendous rate of growth in data can either be a hindrance or a help to organizations looking to gain a competitive advantage from their data and analytics investments. Many believe the only way to deal with the volume of data is to spend endless amounts of time wrangling data from disparate sources, replicating it, bringing it into a single place, and parsing it into bite-sized data sets so it can be analyzed.
Instead, organizations must be able to instantly connect to any data source directly, query, and blend any amount of data. But is it even possible? This section breaks down commonly held beliefs about data management and shows you how to use any data within your organization for better decision-making.
Myth #1
All your data must be in one place to analyze it and get the answers you need.
What’s behind this myth?
The incredible growth in the volume of data an organization collects is met by an equally astounding number of data sources. According to a recent survey, companies draw from an average of 400 different data sources to feed their BI and analytics tools, and 20% of the organizations surveyed reported having 1,000 or more data sources.
A traditional approach to data analytics and BI suggests an organization must bring all of this data together in one place to analyze it. But how? Cloud data warehouses are the approach many organizations use, but time-consuming data preparation, maintenance costs, and data analysis limitations are holding them back.
Companies draw from an average of 400 different data sources to feed their BI and analytics. (Source: IDG Survey)
Why do people believe this?
- Typical BI tools require data to be brought into a single standardized format to ensure consistency.
- Consolidating data into a single place is the only way to use it to enable faster, better decision-making.
- Having data in one place is the only way to see trends and compare data across the enterprise.
Truth: Leave your data where it is.
While there will always be a role for data warehouses featuring highly curated data, volumes are growing exponentially. It is becoming difficult — if not impossible — to keep up with the near-constant requirement to prepare the data for analytics consumption. For your organization to truly get the most out of its data, regardless of how complex your environment is, leave the data where it is and bring your analytics platform to your data instead. To achieve this, look for a platform that can directly query the data, no matter where it is.
Myth #2
You can’t do analytics on large-scale data sets.
What’s behind this myth?
Organizations must capitalize on their explosive data growth. They need an analytics solution capable of handling a large volume of data to do so. But organizations believe they must accept the limitations of BI and analytics tools that rely on in-memory engines to analyze it. These engines are simply not built to handle large data sets. So organizations are instead trained to break up their data and feed it in simplified, bite-sized pieces to the BI engine. Data is extracted and moved elsewhere, whether to Excel or another tool, for transformation before being re-introduced to the BI tool.
Enterprises will generate and manage 60% of the 163 zettabytes of data by 2025. (Source: IDC)
Why do people believe this?
- Most analytics tools are limited to handling data they can access directly from a single data source or data that has been extracted from its original source and blended to create a single data set.
- Analytics tools crash or run slowly when working with large data sets.
- Most analytics tools have data upload limits that require people to break up large-scale data sets.
Truth: You can do analytics in place.
While in-memory engines can handle smaller, less complicated data sets, they can’t keep up with larger ones. So why use in-memory engines? You can do analytics on large data sets with the right type of analytics engine: one that can directly query the data where it is.
Myth #3
An analytics dashboard can only display one source of data.
What’s behind this myth?
In an ideal world, decision-makers across an organization could access a dashboard of all data to gain a true 360-degree look at the trends and factors driving their decision-making. In reality, people have come to expect dashboards that can only display one source of data. So instead of a single dashboard, organizations end up with dozens of dashboard views of all different data sources making analysis and insight difficult to come by.
While this limitation is widely accepted as accurate, what’s also true is that answers to complex questions often require more information from more sources. A common workaround is to extract data from core systems and bring it together in a single dashboard. However, this introduces data latency and replication risks.
“Reports and dashboards are still the most common types of analytics with nearly all organizations (97% and 96%, respectively) indicating they are important or use them currently.” (Source: Ventana Research Analytics and Data Benchmark Research)
Why do people believe this?
- Most BI tools simply cannot integrate data from multiple sources into a single dashboard view.
Truth: You can integrate disparate data into a single dashboard.
Data from one source alone is not always enough. Insights come when data intersect, revealing new perspectives. To see beyond the obvious, you must be able to integrate data from multiple sources, wherever that data resides — Amazon Redshift, SQL Server, Excel, wherever.
The truth is, it’s possible to integrate governed data into a single dashboard with the right platform. With the right decision intelligence platform, any person can display disparate data sources they have managed access to on a dashboard. And better yet, be capable of filtering, slicing, and dicing across that data without duplicating or extracting it to a separate location. That’s where you get unexpected answers.
Myth #4
Data must be replicated and it’s not possible to apply security automatically.
What’s behind this myth?
Many BI tools force people to replicate data from source locations into a data silo or warehouse before they can analyze it. The idea is to copy data from various enterprise sources, clean it, and have a separate, controlled environment to store the data where it can be accessed by the BI tool. In addition to security that is implemented on the data warehouse, some analysts find themselves building content multiple times to go against different data sets to restrict what access is available to the end-user.
Replicating the data preserves the structure of the original source but adds extra steps and complexity to the process as organizations need resources and procedures to maintain the data’s consistency. Is this necessary? Is this smart?
“Data preprocessing such as cleansing and formatting it for analysis is time-consuming. Some estimates suggest that this can account for 80% of the effort in data analysis projects.” (Source: Deloitte)
Analysts spend the bulk of their time on manual tasks such as preparing data for analysis (47%) and checking quality and consistency (45%) in the data rather than doing actual analysis.” (Source: David Menninger, Ventanta Research)
Why do people believe this?
- Organizations are told that replicating data keeps it consistent, reliable, and up to date.
- Typical analytics tools are built to pull from a data warehouse instead of straight from the data source, claiming this improves the speed and efficiency of the data analysis.
- Organizations are encouraged to avoid working directly with source data to preserve it.
Truth: There’s no need to replicate data for decision intelligence.
Data replication is a widely accepted strategy for disaster recovery, but when it comes to analytics, it increases risk. Replicating your data and moving it to another location loses its inherent security, and, depending on the way the data is shared (extracted into intermediate files and shared via email or other insecure means, etc.), can inadvertently introduce additional downstream security concerns (e.g., data getting into the wrong hands). Plus, you risk creating copies of the data that conflict with the source data (data silos). Data latency is another concern: the moment it’s extracted from the source, it’s no longer up to date. Leave the data where it is and avoid the unneeded chaos.
Reactie toevoegen